In our Data & Analytics Thought Leader Series, Datavant’s Head of Data Strategy, Su Huang, interviews leaders who are advancing data-driven use cases at their organization. Today’s interview is with Sid Jain, Head, Global Development, Data Science at Janssen Research & Development, LLC.
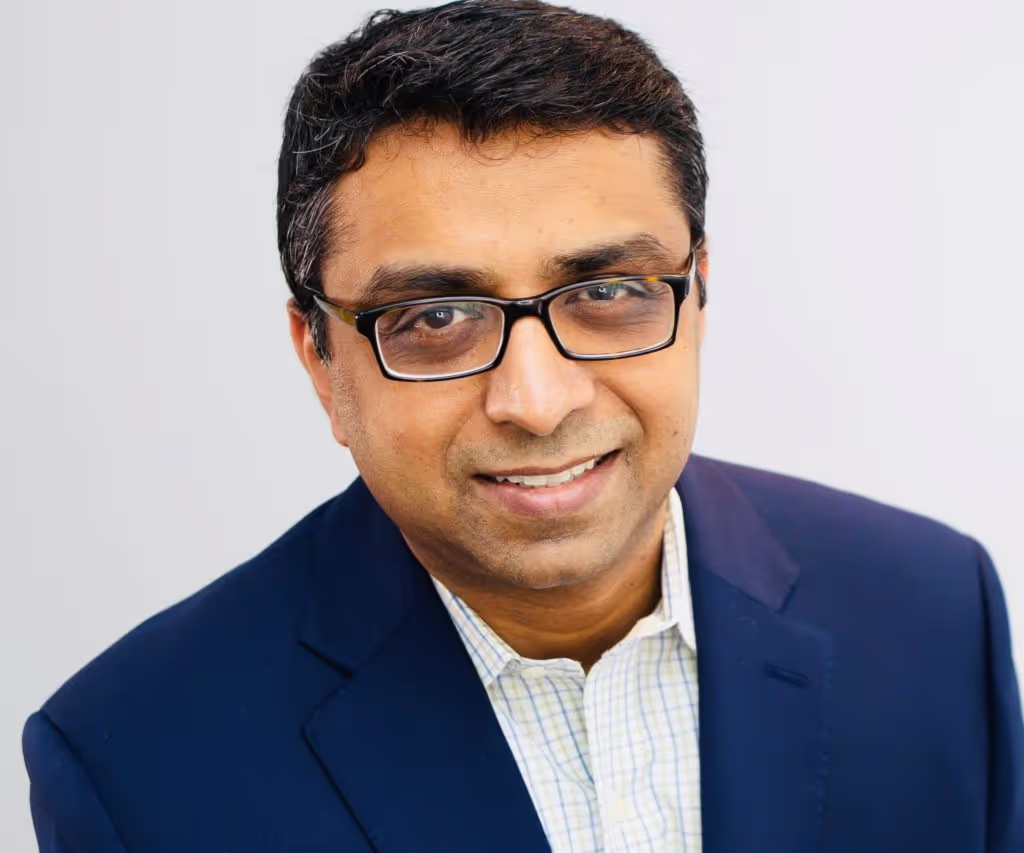
Sid Jain is a leader in using data science applications to advance clinical trial innovation. After starting his career in software engineering, Sid transitioned into healthcare product management, where he played a central role in developing data-driven analytical products and solutions. The span of his 20-year career in healthcare technology has taken him into settings across the entire healthcare landscape, from payors, providers, biopharma, and patients.
At Janssen, Sid and his team are focused on using data science tools and technologies, like artificial intelligence (AI) and machine learning (ML), to accelerate clinical development, with a commitment to not only making drug development faster, better and more efficient — but also making science possible that was not possible before.
Su: Welcome to this interview series with data and analytics leaders in healthcare! Janssen is pretty forward thinking when it comes to how data science, data and analytics can transform pharma R&D. Can you tell us about your team and its core remit?
Sid: In Janssen R&D, our Data Science team is a function that leverages the power of data science, including artificial intelligence (AI), machine learning (ML), real-world evidence (RWE) and digital health, to enhance and accelerate innovation across our portfolio, from early discovery to clinical development and operations.
Within that team, I lead our team that is responsible for using data science in the clinical operations space to drive the clinical development of new medicines and vaccines. One of the foundations of this work is our internal platform, called Med.ai, developed by our Platforms and Privacy team together with Johnson & Johnson Technology and others, which integrates disparate data sources from historical trial operations and real-world data (RWD), such as Electronic Health Records (EHR), claims, labs, and also genomics data.
When we apply data science to this data, we’re able to drive more targeted country and site selection. In particular, genomics data is being incorporated for targeted patient matching in our advances towards personalized medicine — all of it in a manner that puts patients’ privacy first. We also have a strong commitment towards increasing diversity, equity, and inclusion to make our trials representative of the populations we serve, and we are taking a data-driven approach to solving for that.
All of that combined helps us think about trials differently: How are we going about selecting our sites? How can we identify patients that meet inclusion and exclusion criteria for our trials? Is there an opportunity utilize digital / decentralized trial technologies to reduce patient burden and increase participation?
The other area of focus for my team is the linkage of clinical trial data with RWD, or ‘tokenization.’ Our R&D Data Science team, in partnership with our Vaccines team, started using tokenization to link data with our COVID-19 vaccine trial, but it’s really taken off and we are now looking to do this across our portfolio. It’s becoming front and center to our strategy because we strongly believe that linking trial participant data with RWD — with patient consent — can unlock unique insights, especially when you think about long-term follow up while reducing patient burden.
Su: : Having a team focused on using data, analytics and technology to accelerate clinical trials is very ambitious. What was the motivation for Janssen to have a team like this?
Sid: The concept of using data to accelerate clinical operations has evolved over the years — from just relying on personal connections with sites and PIs to using historical operations data and feasibility survey results to pick the right sites for the right trials. Our R&D data science team is taking the next step in what can be done based on emerging technology and data. Today, as more real-world longitudinal data becomes available near real-time, there is an opportunity to apply data science and AI/ML to further improve our ability to predict sites with higher recruitment potential — in addition to continuing to use the historical operations data to predict sites that are likely to default. Ultimately, the motivation for using these tools and technologies is to help bring life-saving and life-enhancing therapies to patients sooner than previously possible.
Su: We definitely see that at Datavant as well, the increasing amounts of different types of data that could be really useful for research. How does your team interact with different functions and stakeholders across Janssen and externally to do this work?
Sid: Our R&D data science team at Janssen partners with all therapeutic areas and functions, as well as with our colleagues in commercial and across our enterprise, in order to ensure we’re leveraging data science to solve the right challenges and drive the greatest impact for patients.
Within my team focused on clinical operations, we work closely with the clinical research and operations teams to inform and understand the study design and make sure that the study can be successful when it is executed. For example, does the inclusion/exclusion criteria accurately reflect the patients that exist in the real world? Are we ensuring that we are in the right places to recruit the right patients — and diverse patients — for the study?
There’s also an external component to how we work. When it comes to data, we need external partners to bring in and source that data. Without high-quality data, there’s no data science. Then there are partners like Datavant — being able to link and tokenize data that creates a longitudinal view across the patient life cycle is critical to serving a variety of use cases.
Su: Turning to the external environment, what are some of the trends you are watching and tracking? How are you seeking to apply them at Janssen R&D?
Sid: COVID-19, for all the bad, did accelerate quite a few of the innovations, such as remote monitoring, tele-visits, and decentralized trials, by several years. There’s still a lot of work to be done from a technology perspective and from an implementation perspective, but I’m very excited for the potential of these technologies to reduce patient burden so that clinical trial research can be more accessible to all patients, especially those from disadvantaged backgrounds.
Natural language processing (NLP) is another key technology that we are investing in. A lot of the times, researchers need details that are only available in unstructured data sources, so either manual human curation or NLP is needed to extract data from doctors’ notes, images, and other non-structured formats — and NLP is much faster and more efficient.
Su: How do you educate internal stakeholders on these capabilities and innovations that your group is identifying and trying to adopt?
Sid: By now I think there’s more or less a wide consensus both at Janssen and in the industry that data science is a core capability that any future-oriented organization needs to invest in, just by virtue of the torrent of data and the value it can generate for drug discovery and drug development. With that said, there is the question of the level of investment and the types of use cases that get pursued. This is where constant prioritization is key, and it becomes really important to bring different stakeholders together and have a joint vision for what you’re trying to accomplish.
Su: What are some examples of how data and data science is accelerating clinical trials? Have there been other tangential impacts from the work that you’re doing that maybe were less expected?
Sid: COVID-19 is a great example of how we used data science for clinical trials. When we started developing our vaccine, we knew we’d need to conduct our trials in places where participants would have high levels of exposure to the virus — so that we could more quickly determine the efficacy of our vaccine. Using a variety of data sources and AI/ML models together with MIT, we were able to predict ‘hot spots’ four months in advance, down to the county or province level, with 90% accuracy. This guided our site selection efforts and enabled us to complete our trial 6-8 weeks ahead of our development timeline, with diverse participation and significant data on emerging variants at the time. Notably, we also used real-world data for long-term monitoring of effectiveness, and linked clinical trial data with real-world data to create the external control arm.
Beyond COVID, we are also leveraging data science to enhance and accelerate clinical trials in other areas across our portfolio, including in immunology and oncology. We’re using it for site selection for clinical trials in highly competitive areas, such irritable bowel syndrome, and in the rare disease space, where it’s difficult to find patients.
Additionally, we were already planning to scale our efforts to improve diversity, equity and inclusion (DEI) in our clinical trials using real-world data, even prior to the recent FDA draft guidance on the topic. With real-world data, linked with race and ethnicity data, we can find and recruit diverse patients into clinical trials, with the goal of ensuring that those trials are representative of the patient population.
Su: What type of organizations do you look to partner with on these kinds of advanced AI and ML applications?
Sid: We don’t think of our partners as vendors, but as collaborators. That requires learning together. In many cases, our partners have mature capabilities and that’s great. But innovation does require experimentation, so we are willing to make an investment in them that is more than the financial investment. We look to make sure that we provide sufficient runway for the experiments and innovations with these partnerships to come through, Ultimately, that innovative DNA and the passion to change the trajectory of human health is key when we select our partners.
Su: Let’s move back to the topic of clinical trials. What do you think is the biggest innovation in this area today? What are the next set of innovations you’d like to see?
Sid: Frankly, innovation is driven by the culmination of data, technology and the ecosystem all coming together.
Why those three? Data is the obvious key ingredient. Technology enables us to bring the data together. I’ll use the example of tokenization technology. It’s great that you have data, but if you’re not able to link those disparate data sources together in a privacy-compliant way that creates a single longitudinal view, it loses its impact significantly. Ecosystem refers to the regulatory ecosystem. Looking at the 21st Century Cures Act, that has been a key enabler to moving the real-world evidence movement forward.
We’re still fairly rudimentary in terms of how patients are diagnosed and how their treatment plan is created. It’s not sufficiently data-driven for the vast majority of patients. So being able to get more data about patients in a secure, compliant way and then being able to both create and deliver more targeted therapies is where my hope is we’re going to be in the next five to 10 years.
Su: What do you think needs to happen in order to see increased use of RWD in regulatory decision making? What are some of the key challenges and bottlenecks that you see in the industry that prohibits that?
Sid: You need broadness of data as well as deeply curated data. The other big part of this is continuous engagement with the regulators. While each study will need to be handled differently, that kind of continuous engagement with the regulators is key to making sure that the data we are using is fit-for-purpose and addresses critical endpoints and research questions.
Su: As connecting different data sets together to create a more longitudinal view becomes more common, how does your organization deal with privacy and compliance issues around the management and use of health data?
Sid: Privacy is paramount to everything we do, even at the cost of taking a step back at times. One of the functions we work very closely with for any use case is our privacy team. Everything we do goes through a privacy review to make sure we’re taking a practical approach to solving for it.
As an example, for tokenization, we took the approach of making sure we were collecting consent before linking trial data with RWD, so the trial participants are fully informed of how their data is going to be used and what safeguards we are putting in place to preserve their data privacy.
Su: How do you see data science today versus its potential in the future and what types of data sets are best positioned to be used for AI applications? What do you think is needed to innovate and accelerate in this area at the enterprise level?
Sid: From a data science perspective, I think we as an industry have come a long way. I won’t say the promise has been met yet, but we’re far from just starting on a journey. We’re in the middle of this journey.
Data science is one of the key pillars of the strategy at Janssen. For us it’s about the promise of precision medicine and realizing its full potential, not just at the end of the spectrum of clinical development but at the beginning. It starts with targeting the right molecules and using AI and ML to drive our discovery process. That is going to be one of the keys to success for data science over the next three to five years.
Su: With that in mind, what do you think will be required to bring about this transformation in healthcare? What does that end state look like to you?
Sid: I look at how the sponsors and regulators are engaging with each other in ways that both protect patient privacy, but also continue to advance innovation. Balancing both is key because we can always take a more conservative view. If we do the right risk-benefit analysis around unlocking the full potential of patient data without making any compromises on the privacy or security of that data, then the potential is enormous over the next five to 10 years.
Su: Thank you so much, Sid, for sharing your perspectives with us today!
If you would like to learn more about this series, please email Su Huang at su@datavant.com.