Introduction
Data will power true transformation in healthcare, revealing insights and making connections that are otherwise unknown. Take the compelling example of multiple sclerosis (MS) where data is going to help redirect efforts to cure and prevent MS. For years, the cause of this debilitating, fatal disease was unknown. Then researchers studied complete patient journeys using the Department of Defense database, a closed system of 20 years of healthcare information from over 10 million service people. This robust data set enabled researchers to find a clear link between Epstein Barr virus and MS making virology a critical path in MS research. Unfortunately, this data set is unique and will not answer every scientific question we face.1
And unfortunately, the way we manage healthcare data today is not setting us up for this type of transformation - or for optimal utilization in general. In fact, a significant amount of valuable healthcare data is not usable, sitting across thousands of siloed sites and databases. The challenge only increases as data multiplies: as patients see more providers, receive more tests, adopt wearables, seek new treatments, and use more devices. Patients also often move between payers and providers, creating a disconnect in their journeys. Compiling these data requires a new way to work with disparate, siloed, and expanding data sets.
The problem isn't just the fact that data is constantly compounding at mind-spinning rates. It is the opportunity cost of the inability to access, use, and glean insights from this data. Data stands in the way of patient care, medical innovations, and so much more.
That’s where data logistics comes in. Data logistics enables the safe, efficient healthcare data connectivity needed for flexible, fit-for-purpose data sets. This paper explores how data logistics has been the missing piece in making data secure, accessible and usable, offering an opportunity to harness the full potential of healthcare data.
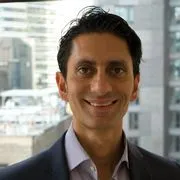
Contextualizing healthcare data within the patient journey
The terms often used to describe the size of digital data can be difficult to comprehend: petabytes, exabytes, zettabytes. Additionally, the digital data universe is growing so quickly, especially in healthcare, that it is on a path to double every 2 years or less.2 Some measures and drivers of this growth include:
- Hospital data: The World Health Forum states that hospitals produce 50 petabytes of data per year3
- Genomics and biomarker data: Genomics and associated biomarker data will potentially generate up to 40 exabytes of data by the end of 20254
- Wearables: Globally, wearable devices are expected to create over 90 zettabytes of information in 20255
For healthcare data, it is easier to illustrate the point by focusing on the patient journey. While this is only a subset of the industry landscape, it is a bit more intuitive than, say, calculating claims data (which in 2022 medical plans executed 14 billion claims-related transactions6).
To do this, we conducted a study using patient-level health data to better explain how each patient’s longitudinal medical history and their experience engaging with the healthcare system are continuously growing larger, longer, and more complex. Starting with how much more information there is per patient, Figure 1 shows how we found that the volume of patient-level records grew 57% in just 4 years.7 This growth reflects how much a patient interacts with the healthcare system. A Centers for Medicare and Medicaid Services study from 2019 found that in 1 year alone, each beneficiary enrolled in Medicare Advantage experienced an average of 21.1 outpatient visits, 3.4 outpatient hospital visits, and 0.2 inpatient hospital visits.8 And that does not account for every interaction they have.
Figure 1. Volume of patient-level records (billions)
CAGR, compound annual growth rate.
Source: Prognos Health data. Valuate and Datavant analysis.
Figure 2. Average records per patient generated per year
Source: Prognos Health data. Valuate and Datavant analysis.
And the number of interactions is only growing, as shown by our study. From 2019 to 2023, per-patient records increased from about 59 to 86 records, even factoring in a decrease during the COVID-19 pandemic (Figure 2).
As Figure 3 suggests, this extra data collection may come from the dramatic increase in the number of provider sources, with a 66% increase in National Provider Identifiers from 2019 to 2023. As all this data grows, so does the risk of duplication and error, with different stakeholders providing and managing information about the same patients. This raises the question: how usable are all these data points?
Figure 3. More providers contributing to patient data pool (provider NPI growth)
Source: Prognos data. Valuate and Datavant analysis.
Even if a patient’s data have been well organized, their next doctor’s visit could upend these efforts. As shown in Figure 4, a single medical event can trigger a vast amount of healthcare data that are independent of one another, captured in separate data sources, and viewed by healthcare professionals in different places. This ultimately leads to potential misunderstandings, misalignment, and missed opportunities for the patient, provider, and other healthcare stakeholders.
Data generated from a single patients office visit
Figure 4. Explosion of data from a single patient encounter - showing how much new data is generated across data sets every time a patient visits a healthcare provider
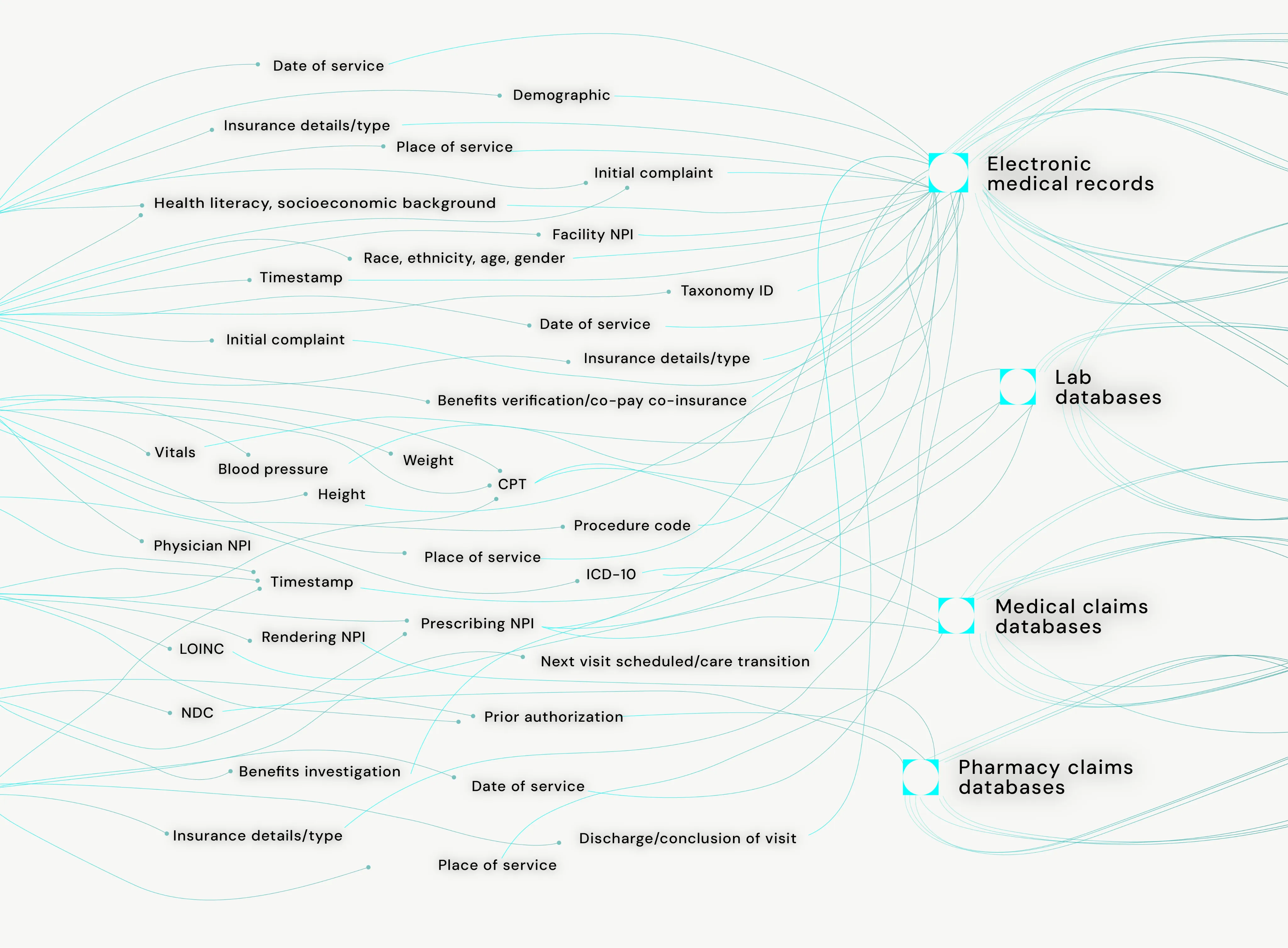
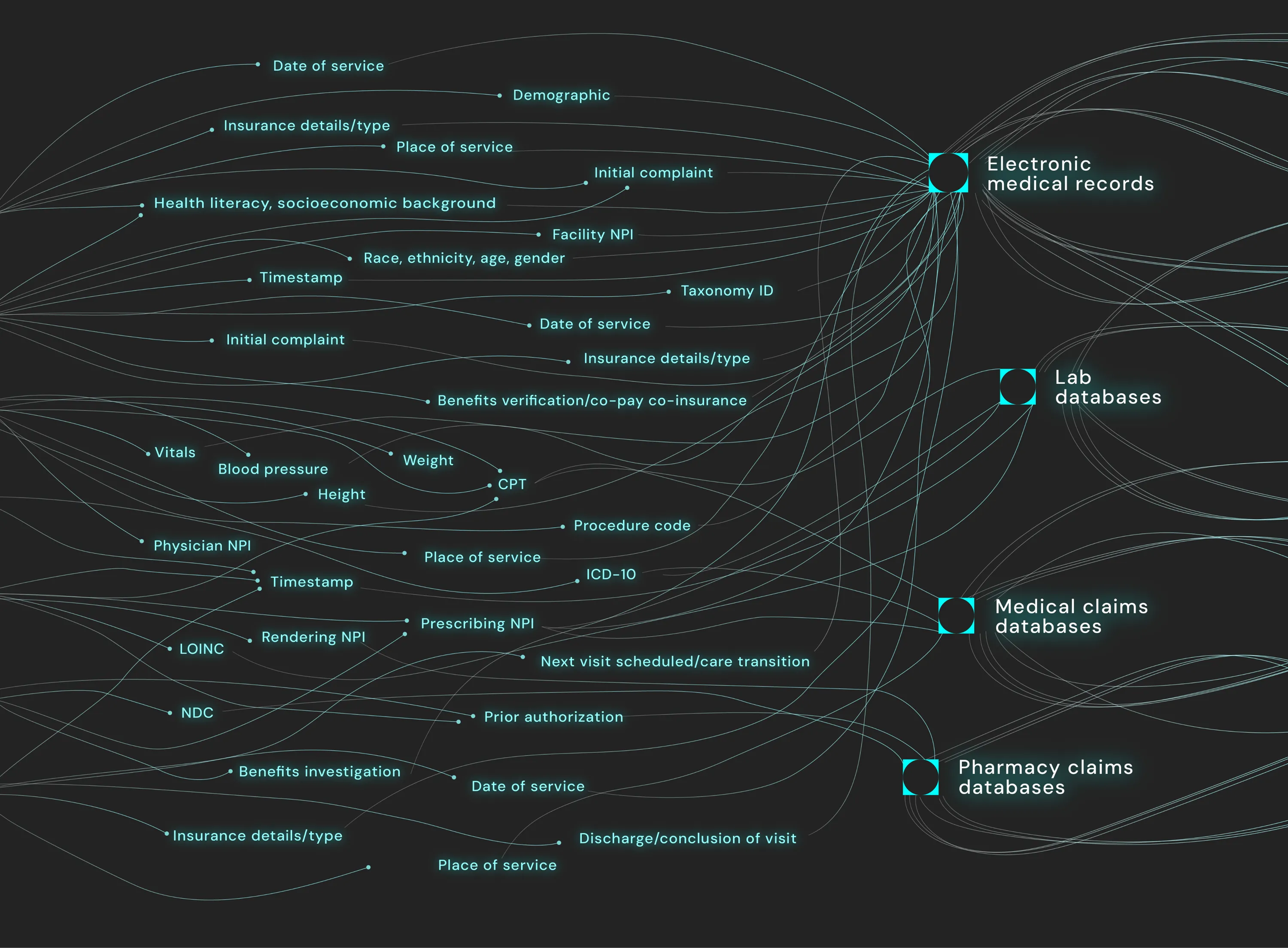
CPT, Current Procedural Terminology; LOINC, Logical Observation Identifiers Names and Codes; NDC, National Drug Code; NPI, National Provider Identifier.
Where healthcare data sit today: A fragmented supply chain
The healthcare data supply chain is complex, fragmented, and unusual (Figure 5). Where other supply chains are designed to move from input to end product, in many ways, the healthcare data supply chain does the opposite. The system is designed to generate, manage, process, protect, and apply data to specific use cases, but it is not designed to move data.
Figure 5. The healthcare data supply chain: sources and estimated value
estimated to be $18.2B in 2023: expected to reach $35.3B in 2028 Rules, protocols, oversights, and systems to ensure data are collected, stored, managed, and used to protect privacy
Estimated value: Healthcare cybersecurity market size alone9
Ways in which health data are analyzed to drive meaningful macro insights
Estimated value: Global healthcare data analytics market size $21.1B in 2021: expected to reach $85.9B by 202710
Ways in which health data are analyzed to drive meaningful macro How data are studied for specific purposes, such as research
Estimated value: Undefined, as governmental and private sources fund this space
The processes and technology that enable data sharing across systems, including the ability to find data sources
Estimated value: Global healthcare data interoperability market size expected to reach nearly $16B by 203011
Ways in which stored data are controlled and accessed to ensure compliance with governance rules,
often including quality controls and formatting (eg, data models to map collected data to ensure it is "seen" correctly)
Estimated value: Global enterprise data management market size expected to grow from $77.9B
in 2020 to $122.9B by 202512
Ways in which data are compiled into databases (eg, paper records, EMRS)
Estimated value: Global EMR market size surpassed $27.42B in 2023 projected to reach $41.87B by 203313
The secure storage of data that have been collected and may continue to be collected
Estimated value: Up to $11T14
Patients generate data, both paper based and digital, through interactions with the healthcare system, services, and technology
Estimated value: Global wearables market size $71.5B in 2022: expected to hit $374.6B by 202215
Source: Prognos data. Valuate and Datavant analysis.
Several key characteristics keep healthcare data “stuck” and hard to use:
Limited interoperability is a multifaceted problem reflecting a lack of standardization by regulators, poor and varied data quality, and limited ability to match patients across providers.17,18 Even at the patient level in a single data management system, an estimated 80% of healthcare data are unusable due to the lack of structure.19 The U.S. government invested $35 billion to encourage the widespread use of electronic health records through the HITECH Act, but the measure failed to substantially improve interoperability of patient records in the United States, highlighting deeper structural deficiencies.20
Patients move through the healthcare system and interact with different providers, each with potentially different data management systems. Their information ends up in silos that are not connected to other institutions if there are no data-sharing arrangements between them. According to Micky Tripathi, National Coordinator for Health IT, Office of the National Coordinator for Health Information Technology, up to 30% of hospitals do not participate in nationwide data-sharing networks.21 Silos can even exist within one system or building. For example, hospitals often keep radiology records in a separate department; thus, access to certain records—even within the same system—may require manual retrieval.22
Protecting patient’s health information is a shared and important priority across healthcare. Regulations, laws (e.g., HIPAA), and other controls exist to ensure that this information is secured and used only for appropriate persons. When records contain protected health information (PHI), data holders such as hospitals and other providers, manage data access with robust, complex systems and processes. While this data governance is critical, it can suboptimize patient care and data usability by slowing down or preventing appropriate data sharing.
Even in cases when PHI is not present, such as using de-identified patient records to construct patient journeys for research, protecting privacy can slow down data sharing.23 Deidentification uses strict privacy and security regulations23 and can influence the way data is structured and stored, as each institution balances concerns over usability, security, scalability, and cost.24,25 This means that data is hard to connect to each other to create a reliable patient journey.
Individuals change providers and health plans so often. Approximately 1 in 5 individuals disenroll from their healthcare plan each year, and 1 in 3 return to the original insurer within 5 years.26 The healthcare provider space is similarly turbulent, with one survey suggesting that 30% of patients selected a new provider in 2021.27 Each time a patient switches health plans or providers, there is no guarantee their historic health data will come with them. It has become evident that the fragmentation of healthcare data is not likely to change. So, how can we begin to think about optimizing access to these data within the existing and new sources, rather than trying to corral data into a single source?
Making your organization’s data management strategy more agile is crucial
There are specific areas where we have seen organizations successfully enhance their data management strategies to help optimize their use of healthcare data:
Recognizing data gaps
Most healthcare stakeholders have their own data sets. The challenge lies in determining how to complement your own data with other sources to help fill key gaps. We recommend a robust data mapping exercise to assess the data that you currently have access to against the use cases you want to support. For example, a payer organization may be underestimating a member’s risk of medical complications because they do not have access to information on new, relevant tests and clinical visits that occurred since the last assessment. A provider might not see an out-of-system urgent care visit that could affect a procedure’s outcome if they only have access to their system’s electronic health records (EHRs).
Working with the right amount of data to fill the gaps
While relying on a single EHR system or data vendor may make data management easier, it leaves organizations with incomplete data. Additionally, trying to access too much data causes inefficient investments. We recommend proactively expanding your data ecosystem in a safe, timely, and cost-effective way through a flexible, federated model. Ensuring that your organization has access to usable data to address relevant gaps does not mean establishing access to every data set available. However, it is important to understand where the desired data sit and to create access to those data as needed via a federated model.
Using technology and expertise to bring data together
Given the increase in health data - often with duplicative information - requires a scalable approach to managing that information while being compliant with varying governance rules. We recommend plugging into a platform that enables you to identify, link, transform, and access the data you need compliantly. This platform can support multiple types of use cases, from linking all a patient’s data to provide a complete patient record to linking billions of records to support the types of analyses that researchers achieved in MS.
Introducing data logistics: The missing part of most data management strategies
To increase the usability of healthcare data, organizations need a strategic and systematic capability to move and protect this information across use cases. That capability is called data logistics. It involves managing information and handling processes optimally, including aspects related to time (flow time and capacity), storage, distribution, and presentation. Effective data logistics helps healthcare organizations improve results while managing costs when capturing, creating, searching, and maintaining data. Fundamentally, data logistics plays a crucial role in addressing healthcare data supply chain challenges by coordinating the movement and handling of data across the supply chain efficiently, allowing compliant data sharing between parties while allowing each organization to maintain control. There are a few key components to data logistics:
Data logistics involves tapping into the larger data ecosystem beyond an organization’s boundaries. Understanding the complete patient record, either identified or deidentified, requires accessing relevant data from various sources. Successful ecosystem access (including governance) and navigation enable data to move between healthcare providers, insurance companies, research institutions, and other stakeholders.
Data logistics leverages a technology platform to optimize workflow related to data movement, storage, and processing. It spans fields such as networking, file/database systems, and process management. Authorized access, as governed by regulations like HIPAA, ensures that patient safeguards are maintained and can often be verified through digital interfaces.
Data logistics ensures that data are handled securely, compliantly, and efficiently. Beyond merely moving data, it focuses on protecting patient privacy and connecting data in a compliant manner. Given that most healthcare data are unstructured, and can even be paper-based, people are often the most effective way of securely and compliantly ensuring data get to those who have a use case ready for the data query. Data logistics cannot exist without people.
Enterprise-grade solutions are critical for your organization’s data strategy
As the leading company bringing data logistics to healthcare, Datavant is dedicated to helping organizations securely and compliantly move health data through our three key pillars of excellence:

We designed Datavant's solutions to optimally address privacy, compliance, and security. We protect health data, while always ensuring every organization has complete control over how their data is accessed and used.

We enable data to move from the very beginning with the broadest footprint of providers in the US. to reach every patient record. We empower organizations by creating access to relevant data sources, so they can put together all the pieces needed for a complete view of the patient.

We deliver data that is relevant and timely. Through technology and our value-added services, we power countless decisions that support clinical, operational and research questions with the most relevant, usable data.
As the leading company bringing data logistics to healthcare, Datavant is dedicated to helping organizations securely and compliantly move health data through our three key pillars of excellence:
Compliant and appropriate sharing of data, both deidentified and identified, to ensure patient privacy.
Working across a variety of systems, such as hospital EHRs, imaging, genomic data, doctor’s visits, and wearables.
Access to structured, semi-structured, and unstructured data.
Functioning in various scenarios, from clinical decisions to research purposes and health plan optimization.
Fig. 6 shows the value of this data logistics approach, highlighting the ability to tap into the broadest data network with a platform designed to support the protection, connection, and delivery of data, regardless of use case. Data logistics can help your organization explore a breadth of new opportunities, from truly understanding all the care a patient receives, to identifying patients who might be at higher risk before they incur prescribed treatments, to finding patients with rare diseases who may be eligible for clinical research access.
Figure 6. The value of data logistics
Exchanging 200 terabytes of data annually
~100M Medical Records/yr, ~100B tokens/month
Prognos Health is a trusted provider of actionable real-world data in the life sciences industry that is driven by its mission to unlock the power of data to improve health. Prognos Health’s exclusive, unique data sets unlock valuable insights in complex clinical populations across the entire commercial life cycle, going beyond traditional real-world data offerings. Prognos helps life sciences companies accelerate the development and delivery of innovative therapies and improve health outcomes by offering fully integrated and harmonized lab and health records on more than 325 million deidentified patients. For more information from Prognos, please reach out to Ashley Triscuit, Marketing Director, marketing@prognoshealth.com.
Valuate Health Consultancy is a consulting firm that combines deep market access and reimbursement expertise, industry-leading data analytics, and robust market research capabilities to address healthcare organizations’ market access needs. Valuate helps healthcare organizations develop bespoke market access strategies by conducting primary and secondary research, deploying advanced healthcare data analytics and engineering, monitoring and assessing health policy changes, developing pricing and contracting strategies, and more. Our goal is to break through market access barriers to help patients get access to the healthcare they need.