It’s been nearly two decades since the National Institutes of Health’s Human Genome Project completed the initial high-quality sequencing of the entire human genome (Nurk et al. 2022). The audacious goal of sequencing and characterizing all human genomic data spanned public and private sectors and crossed national boundaries. As the first 20 years of the genomic revolution come to a close, we are taking a step back to ask, “How far have we come?” and “Where is genomic innovation taking us?”
Countless new initiatives and partnerships have been built to secure and deliver on the promise of genomic data. Excitingly, a number of impressive clinical successes have emerged from these efforts. As expected, genomic innovation reached cancer patients first. Though an oversimplification, cancer is considered a genomic disease. At Memorial Sloan Kettering Cancer Center, physicians and scientists have assembled their gold standard MSK-IMPACT test for tumor-associated genomic alterations (Cheng et al. 2015).
Using IMPACT, physicians can identify critical genetic alterations and identify drugs with the potential to target these specific changes in cancer patients. Thousands of patients have participated in IMPACT-driven cancer care. Importantly, the genomic data from these patients, including outcomes, have been shared publicly via the cBioportal, AACR-GENIE, and OncoKB platforms, which is helping to drive research activities. This achievement was merely a dream in the pre-genomic era.
Perhaps the most profound lesson that has emerged from the genomic era is simply that genetic characterization isn’t sufficient to support novel drug development in most diseases contexts. In a few cancer types, including gastrointestinal stromal tumors (GIST) and pancreatic ductal adenocarcinomas (PDAC) 80% of patients will be scored positive for a critical mutation in the oncogenes c-KIT (Oppelt et al. 2017) or KRAS (Waters and Der 2018), respectively.
These genetic changes are essential drivers in these tumors and treatment is designed accordingly. But in most cancers and other diseases (i.e. cardiac conditions, diabetes, etc.) many other factors contribute to disease development and progression including age and socioeconomic status (Ge et al. 2017). These pieces of the puzzle are not captured by any genomic testing or technology, compelling us to broaden our understanding of human disease and the information we use to develop therapeutic interventions. The last two decades of genomics research and implementation have altered the landscape of human health and disease profoundly, but what will the next decade bring? Based on the current trajectory, we have compiled six predictions on the future of genomic data applications.
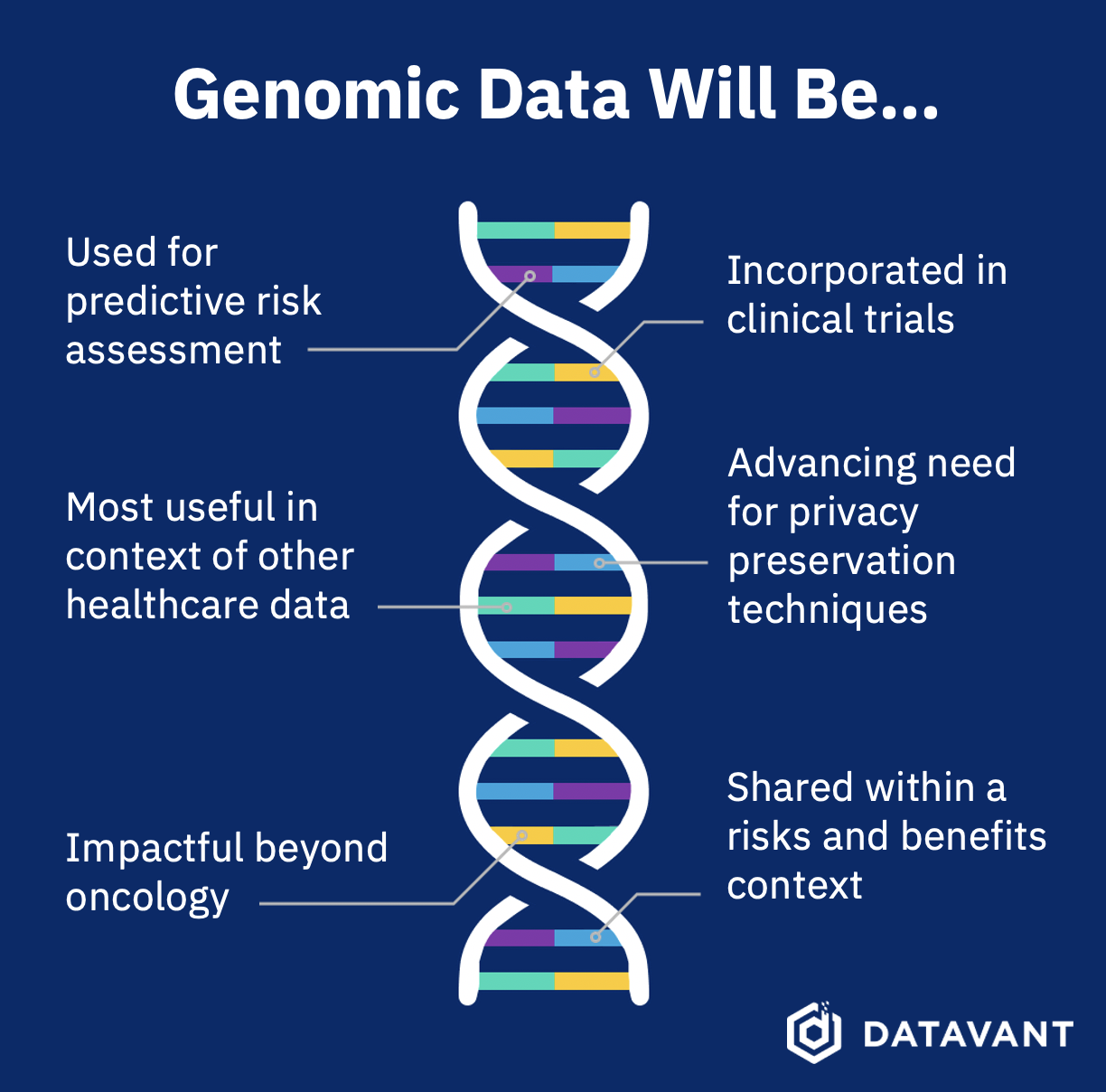
Prediction 1: In some cases, genomic data will fulfill the promise of “Predictive risk assessment” through clinically relevant polygenic scores…
The genome revolution has followed the Gartner Hype Cycle, which estimates response to new technologies over time, beginning with high expectations and transitioning to disillusionment. Ultimately an increase and then plateau in application success occurs as we begin to truly understand the benefits and caveats of something new.
In keeping with this cycle, the human genome project came with inflated expectations. Accordingly, the initial reports from the early 00’s predicted, “profound long-term consequences for medicine” and “now we know what we have to explain” (Lander et al. 2001). These developments were followed by a period of disappointment and confusion about the potential benefits of this achievement. Since 2001 nearly 4000 Genome Wide Association Studies (GWAS) have been conducted. But many of these studies have not been validated or advanced (Mills and Rahal 2019). Generating actionable insights from genome sequencing has proven to be a long journey, and the predictive utility of genomic data has so far been limited to diseases related to only one (or several) genetic changes. However, we’re at an inflection point.
Over the next decade, we’re marching forward on the “slope of enlightenment” wherein researchers will finally be able to validate “genome-wide polygenic scores” to identify profiles of individuals within a population who might be at higher risk for developing a disease. For example, polygenic risk scores have recently been developed and validated for Cardiovascular Disease, to the point where risk assessment accuracy and utility are on par with diseases driven by monogenic (single gene) mutations (such as BRCA mutations in breast cancer (Copson et al. 2018) (Khera et al. 2018). These findings (O’Sullivan et al. 2022) led to the launch of a genetic test for prediction of heart attack risk, developed at the Broad Institute and administered to patients at the Massachusetts General Hospital.
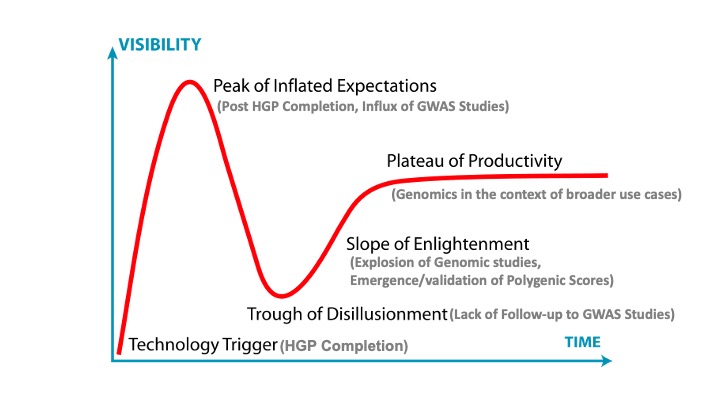
Bottom line: By the end of the decade, we will have risk scores that are validated for clinical decision making in multiple disease contexts.
Prediction 2: … But it’s clear that genomic data will be most useful when combined with other healthcare data.
The genomic revolution helped confirm that most diseases are the result of multiple initiating influences, not limited to genetic alterations. For example, epigenetic changes (changes in gene expression regulation as opposed to changes in gene sequence) have significant influence on disease. Both genetic and epigenetic changes develop with age and due to environmental factors. New technologies have emerged in recent years that are illuminating these aspects of disease including computation pathology, an evolving multi-parameter AI approach to visualizing, characterizing, and analyzing human tissue (Cui and Zhang 2021).
Incorporating genomic analyses into computation pathology will greatly expand the reach of both tools (Schneider et al. 2022). Disease progression is also heavily impacted by access to healthcare and other socioeconomic factors (Boyce et al. 2020; Braveman and Gottlieb 2014). It’s become clear that hereditary, and acquired genomic risk factors should be considered within the larger framework of a patient’s history and health care record, to create the “phenome of a disease”.
The genome-epigenome-phenome relationships are complex but crucial for drug development and clinical trial success. Once we connect these disparate data, we will be better positioned to answer questions like:
- Why does one patient with a BRCA1 mutation get breast cancer, while another does not?
- How does exposure to an environmental factor (e.g., polluted water source) lead to the development or severity of a disease?
- Why do patients with the same tumor and often the same genomic alterations respond differently to identical treatment?
To adequately address these points a patient’s genomic data should be considered in light of their entire medical history (captured in Electronic Health Record (EHR) and medical claims data), laboratory testing (captured in EHR or lab datasets), as well as other sources of healthcare data (such as wearables, socioeconomic data, and others). These sources of healthcare data are also fragmented and require aggregation and linkage.
Bottom line: By the end of the decade genomic data will be connected to EHR, laboratory, socioeconomic, geographic, and other data, to create a longitudinal, holistic view of a patient, and allow for predictive insights into diseases that have complex etiologies.
Prediction 3: Genomic data will deeply impact therapeutic areas beyond oncology.
Perhaps one of the most high-profile initiatives on the heels of the human genome project was the National Cancer Institute’s launch of The Cancer Genome Atlas (TCGA) program, which, starting with glioblastoma in 2008, has funded the sequencing and other molecular characterization of over 33 different tumor types.
TCGA has led to the discovery of novel mutations (e.g., IDH mutations in brain tumors and leukemias, which led to the development and commercialization of enasidenib for Acute Myeloid Leukemia (Stein et al. 2017) ) as well as to better stratification of cancer patients, moving the field of oncology from organ-specific research to molecular profiling and insights.
While oncology is an obvious choice for disease-specific sequencing, there are other diseases that could benefit from insights generated by genome-wide sequencing, especially rare diseases. One of the secondary benefits of TCGA has been the reduction in cost for genome sequencing overall, which makes TCGA-like initiatives more feasible.
Bottom line: By the end of the decade, we will have multiple “TCGA-like” initiatives. TCGA will perhaps become The Comprehensive Genome Atlas, for all diseases.
Prediction 4: Every clinical trial and registry will incorporate genomic data.
Randomized controlled trials are the gold-standard for determining whether an intervention should be approved for treatment in the general population. As therapeutic interventions become more precise, genetic information is becoming part of an increasing number of trials. For example, genetic data is the definitive inclusion/exclusion criteria for many oncology clinical trials (e.g., BRAF mutation status in melanoma),
Roughly 75% of oncology trials and 25% of all trials include genomic assessments (Trzupek and Johnston ) and the FDA has issued guidance on companion diagnostics being developed in parallel with the drug development process. If we agree that the utility of genomic data is increasing across disease areas, especially when analyzed in context of other data, perhaps the most critical application of genomic data will be in clinical trials.
With appropriate patient consent, ideally clinical trials would collect before, during, and after clinical trial completion. The information gathered would be invaluable for future patient cohort identification and interpretation of clinical outcomes.
- Before: support proper randomization. While clinical trial protocols enroll comparable patient populations in their randomized comparator arms, the absence of genomic testing can result in inclusion of confounding genetic variables and muddled trial results.
- During: provide information on genetic responses to drug metabolism and pharmacokinetics in phase 1 studies and contextualize any unexpected top line data
- After: Genomic data could also be a powerful addition to completed trials, especially those where the intervention failed to meet endpoints, as genomic data could help explain super-responder or non-responder populations.
Organizations like the Broad Institute have made significant strides in registry development and utilization, with programs like Count Me In, which registers patients and connects them with scientists working on their specific disease. While incorporating genomic data into a clinical trial poses its own challenges (Joffe et al. 2017), (Mandrekar and Sargent 2009), with time, the opportunities to better understand a novel therapeutic intervention should outweigh the risks.
Bottom line: By the end of the decade, every clinical trial will include a patient-consented option of collecting specimens for genome sequencing, either as part of the trial, or for downstream analysis.
Prediction 5: Genomic data will incentivize innovation in the field of privacy preservation technologies.
In the United States, the Health Insurance Portability and Accountability Act (HIPAA) signed into law in 1996 enforces the privacy of Protected Health Information (PHI) shared by Covered Entities or Business Associates, and allows for the sharing of de-identified health data.
However, genomic data, by virtue of inherited traits, is not easily “de-identifiable”. In addition, publicly available genomic information is making it easier to infer information about an individual’s identity. For example, the Erlich Group’s surname inference study in 2013 showed that by profiling a part of the Y chromosome called short tandem repeats (STR) and searching in genealogy databases that are publically accessible, they could infer likely last names for the individuals profiled (Gymrek et al. 2013).
Another well-publicized example was the risk of re-identification of Dr. Jim Watson’s, co-discoverer of the structure of DNA, APOE gene sequence status (Nyholt et al. 2009). Some APOE variants can contribute to the development of late-onset Alzheimer’s (Green et al. 2009). Unintended dispersal of Dr. Watson’s APOE status would violate his privacy (Wheeler et al. 2008).
Because of the sensitivity of genetic information to the individual and their families, the NIH issued a Genomic Data Sharing (GDS) policy in 2014, which set guardrails and standards around genomic data exchange. Given how important genomic data is to research today and will be in the future, the NIH issued a new Request for Information in 2022 to seek input as it updates its GDS policy for NIH-funded research. This will continue to be an important conversation, on the heels of the shifts happening on the heels of scientific and legislative spheres regarding genetic privacy, covered in more detail from a United States perspective here.
Research on distributions of allele frequency, polymorphisms, and chromosome phenotypes, especially with respect to their intersectionality with geographic and demographic identifiers, could provide a basis for quantifying genetic privacy risk in the future, which may in turn inform bespoke modifications that increase the utility of genetic information in de-identified data while still sufficiently mitigating risk.
In order to safely and securely share and analyze genomic data at scale, privacy preservation may increasingly incorporate additional techniques, including specialized types of encryption, introducing noise through differential privacy methods, federated learning and multiparty computing where data never leaves the original locations, and more (Bonomi et al. 2020) (Almadhoun et al. 2020).
Bottom line: By the end of the decade, new standards will be in place to ensure privacy preservation around genomic data. These standards will support patient privacy in genomics research, and, in turn, help advance privacy techniques for data sharing across the board.
Prediction 6: Genomic data will revolutionize R&D, but some usage will require careful safeguards.
Today, with technological advancements and cost reduction around DNA sequencing, the genomics-based industry is booming. Direct-to-consumer companies are providing tools for genealogical research and health data information in the hands of individual patients. Whereas this wealth of information can help democratize research and development (all the way to citizen science) (Guerrini et al. 2019), it comes with significant risks around data interpretation and data privacy (covered in more detail elsewhere (Bonomi et al. 2020)).
It can also lead to improper data use for discriminatory purposes. In the US, HIPAA (1996) and the Genetic Nondiscrimination in Health Insurance & Employment Act (GINA, 1999) paved the way for the Human Genome Project by providing regulatory frameworks protecting individuals from genetic discrimination. However, there is still room to grow when it comes to patient protection, and to accurate representation of risks and opportunities that come with the increased sharing of genomic data.
To empower R&D while protecting individuals, significant investment in patient education is required (including expanded genetic counseling) and providing informed consent. Ultimately, each individual whose DNA is being sequenced does so at the risk of discovering something about themselves or their families that might cause harm. Beyond education, there will be a push towards responsible sharing of genomic data results back to the individuals who generated the data – the patients themselves, as outlined (Bombard et al. 2019) and (Deignan et al. 2019).
Bottom line: By the end of the decade, there will be increased patient education around the opportunities and risks of sharing genomic data, and patients will have increasing access to their own data.
At the time of the announcement of the completion of the “First Survey of the Human Genome,” President Clinton said “With this profound new knowledge, humankind is on the verge of gaining immense, new power to heal.” What we’ve gained from the publication of the human genome has been nothing short of astonishing. The promise (and challenges) of what’s to come will be equally exciting.
Thank you to Gaurav Singal, MD, Karin Eisinger, PhD, Doug Fridsma, MD, PhD, Su Huang, Joyce Lee, James Gow, David Copeland PhD, Elaine Mitchell, PhD, and Adja Touré, PhD, for their feedback in drafting this article.
References
- Almadhoun, Nour, Erman Ayday, and Özgür Ulusoy. 2020. “Differential Privacy under Dependent Tuples-the Case of Genomic Privacy.” Bioinformatics 36 (6): 1696–1703.
- Bombard, Yvonne, Kyle B. Brothers, Sara Fitzgerald-Butt, Nanibaa’ A. Garrison, Leila Jamal, Cynthia A. James, Gail P. Jarvik, et al. 2019. “The Responsibility to Recontact Research Participants after Reinterpretation of Genetic and Genomic Research Results.” American Journal of Human Genetics 104 (4): 578–95.
- Bonomi, Luca, Yingxiang Huang, and Lucila Ohno-Machado. 2020. “Privacy Challenges and Research Opportunities for Genomic Data Sharing.” Nature Genetics 52 (7): 646–54.
- Boyce, W. Thomas, Marla B. Sokolowski, and Gene E. Robinson. 2020. “Genes and Environments, Development and Time.” Proceedings of the National Academy of Sciences of the United States of America 117 (38): 23235–41.
- Braveman, Paula, and Laura Gottlieb. 2014. “The Social Determinants of Health: It’s Time to Consider the Causes of the Causes.” Public Health Reports 129 Suppl 2 (January): 19–31.
- Cheng, Donavan T., Talia N. Mitchell, Ahmet Zehir, Ronak H. Shah, Ryma Benayed, Aijazuddin Syed, Raghu Chandramohan, et al. 2015. “Memorial Sloan Kettering-Integrated Mutation Profiling of Actionable Cancer Targets (MSK-IMPACT): A Hybridization Capture-Based Next-Generation Sequencing Clinical Assay for Solid Tumor Molecular Oncology.” The Journal of Molecular Diagnostics: JMD 17 (3): 251–64.
- Copson, Ellen R., Tom C. Maishman, Will J. Tapper, Ramsey I. Cutress, Stephanie Greville-Heygate, Douglas G. Altman, Bryony Eccles, et al. 2018. “Germline BRCA Mutation and Outcome in Young-Onset Breast Cancer (POSH): A Prospective Cohort Study.” The Lancet Oncology 19 (2): 169–80.
- Cui, Miao, and David Y. Zhang. 2021. “Artificial Intelligence and Computational Pathology.” Laboratory Investigation; a Journal of Technical Methods and Pathology 101 (4): 412–22.
- Deignan, Joshua L., Wendy K. Chung, Hutton M. Kearney, Kristin G. Monaghan, Catherine W. Rehder, Elizabeth C. Chao, and ACMG Laboratory Quality AssuranceCommittee. 2019. “Points to Consider in the Reevaluation and Reanalysis of Genomic Test Results: A Statement of the American College of Medical Genetics and Genomics (ACMG).” Genetics in Medicine: Official Journal of the American College of Medical Genetics 21 (6): 1267–70.
- Ge, Tian, Chia-Yen Chen, Benjamin M. Neale, Mert R. Sabuncu, and Jordan W. Smoller. 2017. “Phenome-Wide Heritability Analysis of the UK Biobank.” PLoS Genetics 13 (4): e1006711.
- Green, Robert C., J. Scott Roberts, L. Adrienne Cupples, Norman R. Relkin, Peter J. Whitehouse, Tamsen Brown, Susan Larusse Eckert, et al. 2009. “Disclosure of APOE Genotype for Risk of Alzheimer’s Disease.” The New England Journal of Medicine 361 (3): 245–54.
- Guerrini, Christi J., Meaganne Lewellyn, Mary A. Majumder, Meredith Trejo, Isabel Canfield, and Amy L. McGuire. 2019. “Donors, Authors, and Owners: How Is Genomic Citizen Science Addressing Interests in Research Outputs?” BMC Medical Ethics 20 (1): 84.
- Gymrek, Melissa, Amy L. McGuire, David Golan, Eran Halperin, and Yaniv Erlich. 2013. “Identifying Personal Genomes by Surname Inference.” Science 339 (6117): 321–24.
- Joffe, Erel, Alexia Iasonos, and Anas Younes. 2017. “Clinical Trials in the Genomic Era.” Journal of Clinical Oncology: Official Journal of the American Society of Clinical Oncology 35 (9): 1011–17.
- Khera, Amit V., Mark Chaffin, Krishna G. Aragam, Mary E. Haas, Carolina Roselli, Seung Hoan Choi, Pradeep Natarajan, et al. 2018. “Genome-Wide Polygenic Scores for Common Diseases Identify Individuals with Risk Equivalent to Monogenic Mutations.” Nature Genetics 50 (9): 1219–24.
- Lander, E. S., L. M. Linton, B. Birren, C. Nusbaum, M. C. Zody, J. Baldwin, K. Devon, et al. 2001. “Initial Sequencing and Analysis of the Human Genome.” Nature 409 (6822): 860–921.
- Mandrekar, Sumithra J., and Daniel J. Sargent. 2009. “Genomic Advances and Their Impact on Clinical Trial Design.” Genome Medicine 1 (7): 69.
- Mills, Melinda C., and Charles Rahal. 2019. “A Scientometric Review of Genome-Wide Association Studies.” Communications Biology 2 (January): 9.
- Nurk, Sergey, Sergey Koren, Arang Rhie, Mikko Rautiainen, Andrey V. Bzikadze, Alla Mikheenko, Mitchell R. Vollger, et al. 2022. “The Complete Sequence of a Human Genome.” Science 376 (6588): 44–53.
- Nyholt, Dale R., Chang-En Yu, and Peter M. Visscher. 2009. “On Jim Watson’s APOE Status: Genetic Information Is Hard to Hide.” European Journal of Human Genetics: EJHG 17 (2): 147–49.
- Oppelt, Peter J., Angela C. Hirbe, and Brian A. Van Tine. 2017. “Gastrointestinal Stromal Tumors (GISTs): Point Mutations Matter in Management, a Review.” Journal of Gastrointestinal Oncology 8 (3): 466–73.
- O’Sullivan, Jack W., Sridharan Raghavan, Carla Marquez-Luna, Jasmine A. Luzum, Scott M. Damrauer, Euan A. Ashley, Christopher J. O’Donnell, Cristen J. Willer, Pradeep Natarajan, and American Heart Association Council on Genomic and Precision Medicine; Council on Clinical Cardiology; Council on Arteriosclerosis, Thrombosis and Vascular Biology; Council on Cardiovascular Radiology and Intervention; Council on Lifestyle and Cardiometabolic Health; and Council on Peripheral Vascular Disease. 2022. “Polygenic Risk Scores for Cardiovascular Disease: A Scientific Statement From the American Heart Association.” Circulation, July, 101161CIR0000000000001077.
- Schneider, Lucas, Sara Laiouar-Pedari, Sara Kuntz, Eva Krieghoff-Henning, Achim Hekler, Jakob N. Kather, Timo Gaiser, Stefan Fröhling, and Titus J. Brinker. 2022. “Integration of Deep Learning-Based Image Analysis and Genomic Data in Cancer Pathology: A Systematic Review.” European Journal of Cancer 160 (January): 80–91.
- Stein, Eytan M., Courtney D. DiNardo, Daniel A. Pollyea, Amir T. Fathi, Gail J. Roboz, Jessica K. Altman, Richard M. Stone, et al. 2017. “Enasidenib in Mutant IDH2 Relapsed or Refractory Acute Myeloid Leukemia.” Blood 130 (6): 722–31.
- Trzupek, Karmen, and Jill Johnston. n.d. “[No Title].” Accessed July 25, 2022. https://medcitynews.com/2018/12/adding-genetics-to-the-clinical-trial-ecosystem/.
- Waters, Andrew M., and Channing J. Der. 2018. “KRAS: The Critical Driver and Therapeutic Target for Pancreatic Cancer.” Cold Spring Harbor Perspectives in Medicine 8 (9). https://doi.org/10.1101/cshperspect.a031435.
- Wheeler, David A., Maithreyan Srinivasan, Michael Egholm, Yufeng Shen, Lei Chen, Amy McGuire, Wen He, et al. 2008. “The Complete Genome of an Individual by Massively Parallel DNA Sequencing.” Nature 452 (7189): 872–76.
Editor’s note: This post has been updated on December 2022 for accuracy and comprehensiveness.