Special thanks to the following individuals for their help writing this article: Surafeal Asgedom, Michael Stebbins, David Shulkin, Niall Brennan, Tom Carton, Andrew von Eschenbach, and Charlie Rothwell.
***
In the US, there are over 2,000 government health data sets at the federal, state, and local levels, including several of the largest surveillance databases (such as FDA’s Sentinel and the CDC’s surveillance infrastructure) and one of the world’s largest claims databases (CMS data) and one of the largest electronic medical records datasets (VA data). Historically, government data has been created, stored, and analyzed in data silos. This fragmentation limits the utility of government data in answering critical public health questions. This article provides an overview of the landscape of government health data sources as well as the opportunity for a more effective public health infrastructure.
***
One of the challenges the COVID-19 pandemic has highlighted is the level of health data fragmentation in the United States. Millions of patients have had the disease, and vaccination efforts are underway, yet as a society we still struggle to understand fairly basic questions about the epidemiology of the disease, the long-term impact of the disease, the efficacy of various public health interventions, and the impact of the vaccine. For example, given the amount of data that has been collected at this stage, as a society we should be able to answer:
- What is the impact of opening or closing schools on the spread of the disease?
- What factors influence how severe a case of COVID-19 is for an individual patient?
- Are new mutations more virulent with children?
Going forward, as the vaccine is more widely distributed, questions about the efficacy of the vaccine, and the long-term impacts of the pandemic on both individuals and public health, should be able to be answered:
- What is the vaccine’s impact on transmissibility?
- Have changes in access to medical care during the pandemic resulted in higher rates of preventable disease?
- What are the demographics of vaccine recipients?
- Is the vaccine safe for pregnant women?
- How high is the prevalence of long COVID-19 patients, and are there any interventions effectively treating this syndrome?
The challenge of data fragmentation doesn’t just apply to COVID-19, but to all diseases. There is a tremendous opportunity to use data to improve healthcare across diseases. Every time patients see a doctor, visit a pharmacy, check into a hospital, take a lab test, or pass away, there is information collected about the safety and efficacy of drugs, the epidemiology of diseases, and the health of populations that should not be lost. However, all of these disparate data points have limited utility when analyzed individually — it is when they are brought together that these data points form a full picture of the patient’s health. Each additional piece of data that can be linked together has the potential to exponentially increase the value of the data set for understanding key public health questions.
Thus, linking data across these silos is critical to solving the larger health problems that still plague us: expensive and redundant care, poor health outcomes (especially for disadvantaged populations), poor care coordination across agencies (especially when crossing state and Federal boundaries of responsibility), poor public health visibility, poor coordination of social benefits to those who need them to prevent health problems, and many more.
We’ve written extensively about how data is fragmented in the commercial data ecosystem, and created a map of the commercial data ecosystem. In this post, we focus on the challenge of data fragmentation at the government level. On top of the complexity of the commercial data ecosystem, there are more than over 2,000 data sets across federal, state, and local governments that incorporate different types of health information, ranging from lab test results to drug safety surveillance to data on socioeconomic determinants of health – and thousands more from NGOs, universities, and institutions that work closely with the public sector. Yet for all this data that is collected, there is very little connectivity across parts of the government, either at the federal, state, local, or tribal level.
Below is a sampling of government agencies and subagencies with health relevant data. This graphic is not comprehensive, and includes sample state and local agencies along with federal agencies, initiatives, and purpose-built datasets.
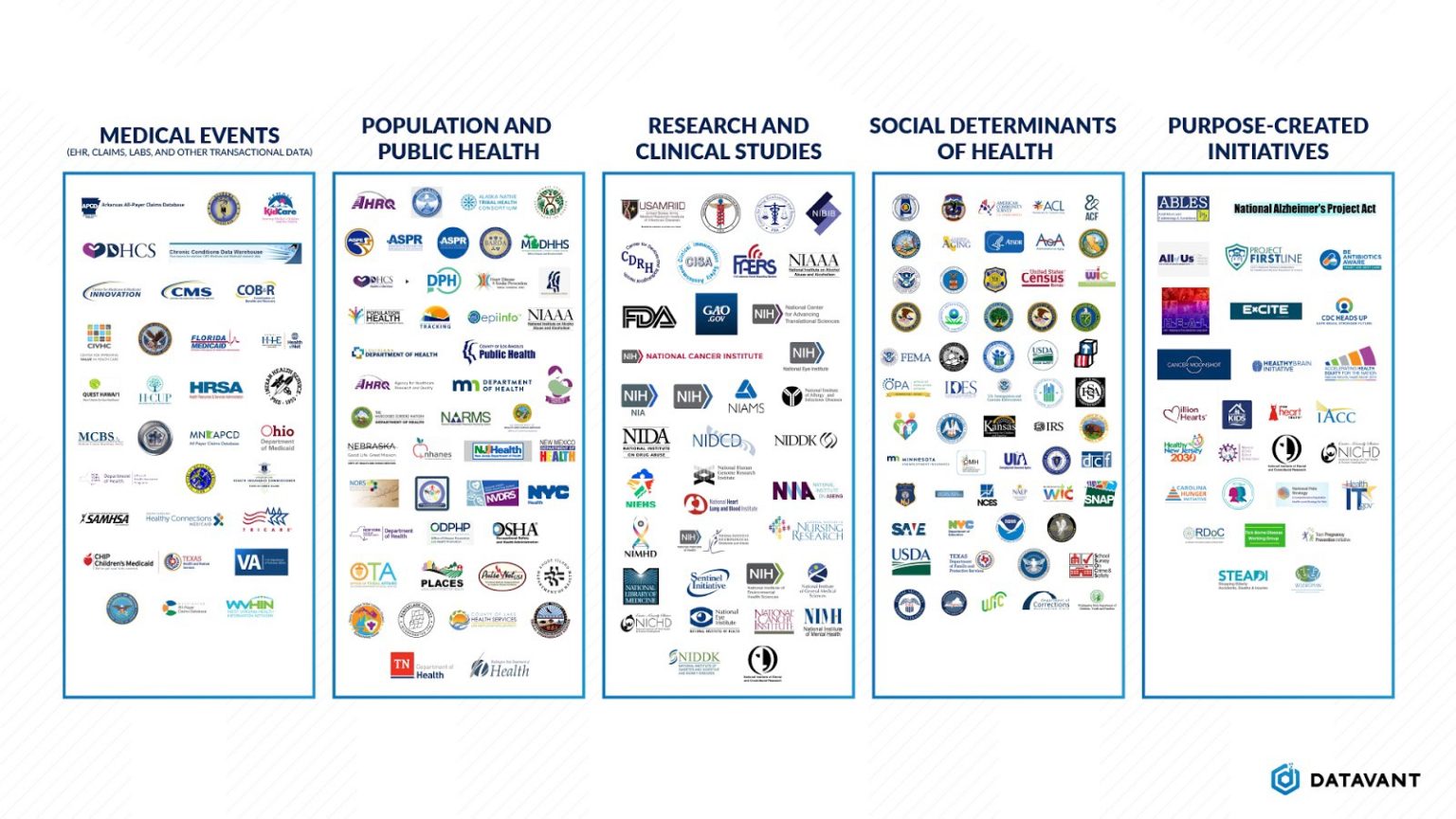
How Data Silos Emerge
Each of the government agencies, subagencies, and initiatives listed above has its own goals, operational processes, and funding, and has developed operational processes to fulfill its individual mission. This specialization creates data silos, as well as duplicative data collection when different agencies have similar questions to answer.
Take for example Jane, a 70 year old living in a skilled nursing facility. When Jane receives a COVID-19 vaccine, that piece of information is captured by numerous different federal, state, and local agencies, each for their own purpose:
- Jane’s local public health department tracks vaccination rates to understand the vaccine rollout effort in the local community, especially in high risk populations.
- Medicare (CMS) receives the claim for the vaccine in order to process payments to providers. If Jane is dual-enrolled in Medicaid, her state Medicaid program may also receive a claim for the vaccine or related services.
- The CDC tracks Jane’s vaccine to understand trends in vaccination and infection rates.
- The Agency for Healthcare Quality and Research (AHRQ) tracks vaccination rates within institutional care settings in order to understand care quality.
- State governments may have their own regulatory bodies for assisted living facilities, which track vaccination rates to understand care quality at those facilities.
- The FDA’s Sentinel group will monitor the health outcomes of vaccinated patients to spot any early safety concerns with the new vaccines.

In this example, Jane’s vaccination status is relevant to at least five government agencies, but each collects the data separately as part of its operational processes or to support its own analytics. Each agency has invested in data collection, but still has an incomplete picture of Jane’s health. For instance, the CDC can track vaccination rates, but does not have information on whether Jane later receives treatment for the virus, which is a data point held by CMS.
Historically, government agencies have created specialized initiatives focused on a single disease area or public health issue to address this issue of redundancy and fragmentation. For example, to better understand the disparate impacts of COVID-19 on minority populations, the CDC began collecting data on patient ethnicity in August 2020, five months after the beginning of the pandemic. However, to preserve patient privacy, these initiatives collect only the minimum necessary information to fulfill their specific purpose. That mindset results in creating yet another data silo, custom-built to answer another limited set of questions.
Power of Linked Data
Individual agencies or initiatives can help answer specific questions and solve problems in the immediate term, but the challenge is to respond to pan-health care questions in which no single data set is sufficient to support decision-making. For these “big questions”, data sets must be linked together to see the entire patient journey, inclusive of the environmental, social, and genetic factors that led to disease onset, through the entire care-path, to the long term outcomes for that patient.
To have a holistic view of a patient and their experiences, researchers need to be able to link the disparate data silos at a patient level, without compromising patient privacy. Patient privacy is a key challenge to data linkage because organizations are reluctant to share identified information (Protected Health Information) with other entities, even when they are other government agencies. To make these data exchanges more acceptable, institutions should consider whether to de-identify data before sending it; emerging cryptographic technologies in the domain of “privacy-preserving record linkage” can allow data to be linked while privacy is protected.
Expanding the use of data linking would enable the government to better understand our healthcare system and its delivery patterns, even beyond COVID-19. For example, duplicative provision of care may cost the U.S. healthcare system up to $78 billion a year. Linked data would enable the government to identify the types of services, both medical and social, that are most likely to be unnecessarily duplicated as patients move between disparate agencies.
Below, we’ve highlighted three sample areas where linked data could enable researchers to answer questions related to COVID-19’s impacts, oncology, and the opioid epidemic, and could enable the government to more effectively deliver interventions:
COVID-19 Research
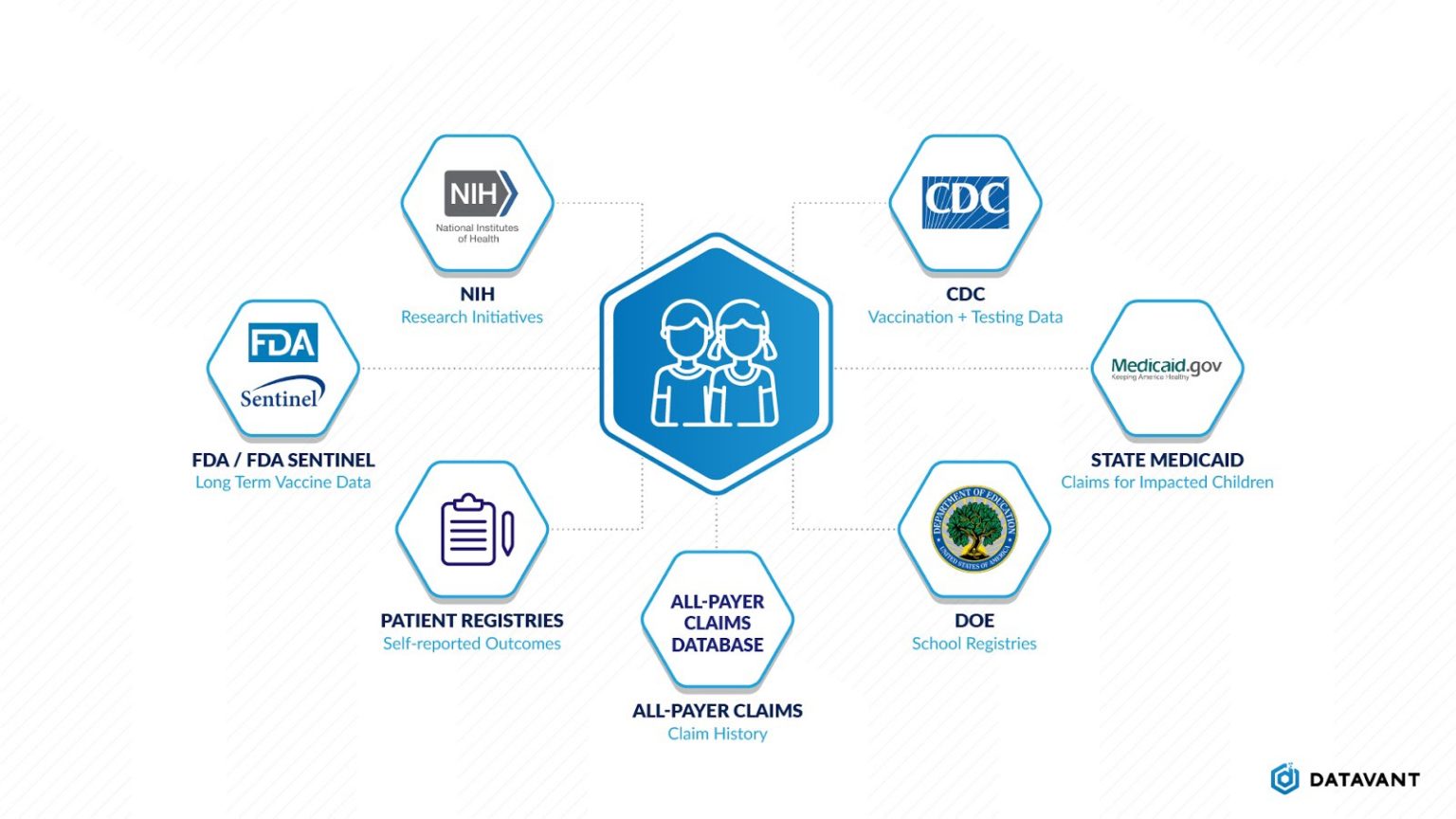
Linked data can be used to understand the long-term impacts of COVID-19 on patients. For example, one impact of COVID-19 in children is multisystem inflammatory syndrome (MIS-C). The long-term impacts of this syndrome are still unclear, but numerous data sources will capture relevant information:
- The CDC has infection and vaccination information, showing which children were infected and which will have received the vaccine
- The Department of Education has school registration information to understand how children have come into contact with each other and may have spread the virus to each other
- State Medicaid programs will have claims history for some children, both at the time of infection and until the child leaves the Medicaid program
- CMS has data on Medicaid coverage, and some all-payer claims data sets will have longitudinal claims data for children
- Patient registries have self-reported synonyms on patients
- The FDA has data tracking the long-term impacts of the vaccine
Similar data sources can also be used to understand the impacts of long COVID in adults. Additional relevant data sets for long COVID could include:
- Social Security Administration (SSA) data on which individuals take long-term disability leave due to long COVID or related disorders
- State unemployment offices have data on changes in workforce participation patterns among populations impacted by long COVID
- Tricare, the Department of Defense, and the VA have claims data on adult populations seeking treatment to help with the impacts of long COVID
Opioid Epidemic

Similarly, key questions about the opioid epidemic can be answered by linking together data sets across multiple agencies:
- The Department of Justice and Bureau of Prisons have data on the prevalence of opioid addiction in correctional settings, as well as encounters with the justice system
- The National Institute of Drug Abuse within the NIH has data tracking the epidemic
- The National Center for Health Statistics (NCHS) has mortality data that can be used to understand how the epidemic has impacted mortality rates for different populations
- State and local public health agencies have tracked the epidemic in their local communities, as well as have records on which interventions were taken at what time
- The CDC has overdose surveillance information
- Medicare and Medicaid claims data shows prescription patterns for prescription painkillers, as well as what other medical services people impacted by addiction have sought
- Socioeconomic determinants of health data from numerous different agencies can illustrate how underrepresented and socioeconomically disadvantaged groups have been disproportionately impacted. For example, the Department of Labor and state unemployment boards shows how employment trends have changed for individuals and areas impacted by the epidemic.
- Agencies such as the Department of Housing and Urban Development and the Health Resources and Services Administration can use this linked data to ensure that services such as supportive housing are being delivered to the right individuals at the right time
Cancer Research

The Cancer Moonshot was designed to accelerate research into new therapies for cancer, as well as improving early detection and prevention of cancer. Data linking would enable future initiatives like the Cancer Moonshot to answer key questions about therapy effectiveness and cancer prevention:
- CMS and the VA have claims data for individuals receiving military healthcare, Medicare, or Medicaid services. These claims show the medical services that patients received. In addition, all-payer claims databases at the state level contain claims on Medicaid and commercially insured patients
- The FDA has clinical trial readouts and other data from clinical trials, which can be linked with claims data and other data sets to identify characteristics of patients who may be super responders or more likely to have adverse events
- HRSA and the Indian Health Service have data on the healthcare received by and health outcomes of underrepresented groups, who are less likely to be participants in traditional clinical trials
- NCHS has mortality data, which is crucial for understanding patient outcomes
- The IRS, as well as the Department of Labor, Department of Education, the Department of Housing and Urban Development, and many others, have data on the socioeconomic determinants of health that may influence a patient’s likelihood to develop cancer and access the appropriate treatment
- The Environmental Protection Agency has data on environmental hazards that may increase an individual’s chance of developing cancer
Realizing the Power of Public Health Data
The gaps in today’s system have been made clear by the COVID-19 pandemic. The inability for the CDC to link data across state public health agencies impeded the CDC’s ability to create dashboards to understand case loads across various geographies. Instead, the Johns Hopkins COVID-19 dashboard became the authoritative source for COVID-19 case numbers, as it efficiently aggregated the disparate state-level data silos.
To be clear, these gaps are driven by the inability to link data rather than gaps in data collection efforts. Each government agency and initiative has made foundational investments in collecting and understanding data in order to provide the most effective and efficient services to its constituents.
Today, this data collection at the federal level is governed by the Paperwork Reduction Act, which requires federal agencies to develop information collection requests for any data gathering process. As a result, the federal government has an inventory of data sets that have been collected over time and can easily analyze which data sets should be connected to one another. Connecting these data sets would also reduce the burden of data gathering activities on the public, one of the main goals of the Paperwork Reduction Act.
The next step to unlocking the power of this data is to link it across data sets in a privacy-protecting manner, which will enable researchers and policymakers to answer basic, foundational questions about how to best provide healthcare services today. Privacy-protecting data linkage across data sets will enable researchers to connect existing data sets to answer pressing questions with minimal need for additional data collection, and ensure that patients receive the benefits of linked data without compromising their privacy.
Public institutions have taken the first steps towards unlocking the power of linked data. For example, N3C, the National COVID-19 Cohort Collaborative, is linking data from disparate clinical sites to speed research into the COVID-19 pandemic. The All of Us program has linked data across fragmented EHR records to understand which types of patients are more likely to receive fragmented or incomplete care.
If more government institutions make their data linkable with other government institutions, we can dramatically increase the speed at which researchers can find answers to questions about the COVID-19 pandemic. But the power of linked data is not confined to this pandemic; instead, linked data can be used to ensure that patient cohorts are well understood in their complexity, so that targeted and meaningful interventions can be made to improve public health for the many chronic conditions prevalent in the United States. Solving the government health data fragmentation challenge, while still protecting patient privacy, will dramatically improve patient outcomes across the United States and the world.
¹ https://www.thelancet.com/journals/lancet/article/PIIS0140-6736(20)32220-0/fulltext
² Pew Charitable Trusts: https://www.pewtrusts.org/en/research-and-analysis/articles/2019/12/09/improved-provider-coordination-can-reduce-health-care-cost
³ https://pra.digital.gov/burden/