
Launched in 2020, the COVID-19 Research Database (informally, the RDB) began as a partnership of more than a dozen not-for-profit and commercial health data organizations with a desire to pool resources to fight the COVID-19 pandemic. Using Datavant’s data de-identification and tokenization, the collaborating organizations tokenized their cohort data – including electronic health records (EHR), claims, social determinants of health, long-term post-acute care, and specialty disease data – and contributed it to a centralized repository where academic researchers could apply for access to study the impacts of COVID-19.
The monumental RDB infrastructure was stood-up in 6 weeks in 2020 and immediately pushed the envelope of linked health data. Prior to the RDB, there existed no other centralized repository containing data from such a broad variety of sources, on more than 300 million patients, completely de-identified, in which tokens enabled linking.
Revolutionary studies in patient outcomes via the RDB
A year ago, we highlighted a handful of the groundbreaking studies built off of the RDB. Our original aim in writing this post was to highlight more of the groundbreaking studies that have emerged from the RDB, but there are now too many (at least 60 so far) to discuss in a short blog post. A large collection of these studies are available at the Health and Human Services Technology Group (HTG) website, and yet more studies have appeared in the National Bureau of Economic Research (NBER) portal.
Studies like these were either impossible or extremely rare before the RDB.
All of these studies involved Datavant’s tokenization technology, and many of these studies were not possible to execute before the creation of the RDB. For example, Engy Ziedan et al. investigated the mortality effects of healthcare supply shocks. To their knowledge, this was the first health economics study making use of a linkage between EHR data + mortality data. Another first is Samer Kharroubi and Marwa Diab-El-Harake’s paper on sex differences in COVID-19 diagnosis published in Frontiers in Public Health.
Studies like these were either impossible or extremely rare before the RDB for several reasons. Most academic researchers do not have the opportunity to work with real world data because it is both expensive to obtain and often siloed between companies and organizations. Research grants are often time-restricted and limited in scope, so academics sometimes hesitate if a study necessitates learning a new framework or tech stack. Further, even if a researcher has access to the necessary datasets, the challenge of analyzing tokenized data and developing a custom matching algorithm for comparing datasets with tens of millions of records and containing a variable number of tokens per record can prove prohibitive to conducting research.
To further complicate this scenario, personally identifiable information (PII) is in constant flux within datasets: patients move, change their names, and undergo gender transitions. When an individual’s PII changes, the tokens within a dataset change accordingly with these fluctuations. Many academic researchers simply do not have the support of an engineering team to manage this kind of work on top of performing the actual study.
Comparing records before Datavant Match
Connecting the world’s health data to improve patient outcomes has been Datavant’s mission from the outset, but the urgency of the global pandemic accelerated the need for faster, more robust research. The creation of the RDB was a monumental step in connecting many data silos, solving one of the biggest problems preventing such large scale studies. For Datavant, the RDB spotlighted the value of the sophisticated privacy preserving record linkage technology of Datavant Match. The RDB became a proving ground, a real-world use case for us to demonstrate the effectiveness and efficiency of our technology for linking real world data (RWD) and to enable new horizons of research.
Prior to Datavant Match being incorporated into the RDB, Datavant took in cohort data, generated a set of tokens based on different fields of underlying PII, and returned the cohort data back to the customer with tokens applied.
Below is a simplified overview of our token generation process, followed by a pair of records with tokens attached to them.
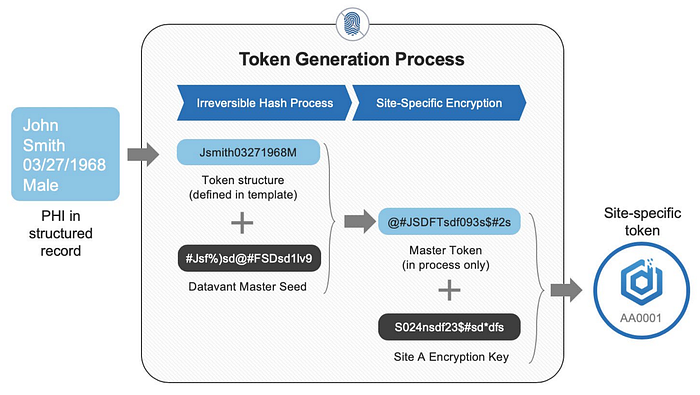
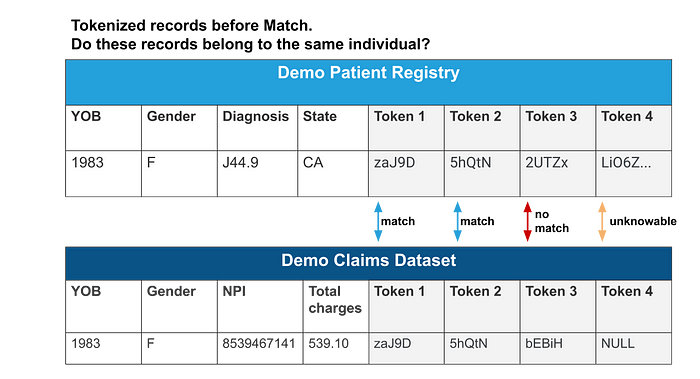
Tokenized records before Datavant Match
Learn how Datavant generates tokens.
A researcher then needed to develop their own algorithm and code to compare records across linked datasets within the RDB to determine if, for example, the two records shown above belonged to the same individual. In reality, a researcher might work with a larger number of tokens than the 3 or 4 tokens shown above.
A clear signal within noisy data
It would be obvious (and perhaps silly) to state that a large, tokenized dataset contains a lot of information, but it’s worth remembering that not all of the information in such a dataset will be relevant to every potential use case that could be informed by that dataset. For the RDB to be fully functional for the widest body of researchers regardless of their engineering abilities, the burden of record matching needed to be removed from users’ shoulders. Datavant Match provides a way to cut through the “noise” of a large dataset and get to meaningful observation faster.
Datavant Match is built on a probabilistic machine learning algorithm trained on over 6 billion real-world record pairs that harmonizes, de-duplicates, and links data. Match analyzes the tokens and certain pass-through fields applied to each record to generate a unique Datavant Identifier. This unique identifier allows a researcher a vastly simplified means to determine if two records containing different PII (and therefore different tokens) do or do not belong to the same individual. Below are the same two example records shown above. They have been determined by Datavant to be a match as shown by their matching Datavant ID.

Datavant Match employs our new generation of tokens, which use sophisticated name and address standardizations, allowing us to match records even if they contain typos in the PII.
With Match, an interested researcher no longer needs to devise their own means to compare many columns of tokens in order to determine if multiple records match a single individual. It also further eliminates the need to worry about why one dataset may only have 2 tokens applied to it and another dataset may have 3 or 4 tokens applied to it.
Users can also configure the target precision and recall of Match based on use case, changing the linking threshold. Certain use cases may prefer higher precision at the cost of recall, and vice versa.
High precision (i.e., higher threshold for determining a match) is useful in research-grade use cases, allowing customers to minimize false-positive matches.
Examples include:
- External control arms
- Clinical trial recruitment
- Public health studies
- Real-world evidence for indications and long-term safety studies
High recall (i.e., lower threshold for determining a match) works well for commercial analytics and health economics and outcomes research studies. This setting decreases false negatives matches.
Examples include:
- Burden of illness
- Comparative effectiveness studies
- Cost-effectiveness analyses
- Long-term observational studies
You can read elsewhere on this blog where we have investigated some strategies for comparing records and adjusting for precision and recall.
(Incidentally, now that Datavant Match is available as part of the RDB, research teams who have already published on RDB data are planning follow-up studies comparing their own matching algorithms with Datavant’s technology, which could perhaps be considered a new form of self-peer-review!)
Preserving privacy while linking data
All of the datasets going into the RDB must be certified as de-identified. The Datavant ID is a highly-secure, encrypted key that preserves privacy while enabling patient linkages. Within the RDB, researchers can choose to access datasets that stand alone (unlinked) or datasets that are already linked. In the case of unlinked datasets, the Datavant ID can be used as a de-duplication tool, or can be used as a tool to determine if a particular dataset is useful for the specific study. Because linking datasets necessarily increases the possibility of PII being tied to a specific individual, linked datasets have undergone more in-depth remediations on their data. Or approached from the other side, unlinked datasets necessarily offer more fields of information around which to conduct research. Datavant’s Privacy Hub provides expert determination for all of the linked data sets within the RDB.
We’re still just scratching the surface of potential insights.
Revolutionizing research and improving patient outcomes
Datavant Match has already powered many studies beyond the RDB. Some of these studies have been catapulted into the national media spotlight for the types data they have compared. In particular, a team from Yale published an NBER working paper connecting mortality data and voter records, a novel study which was featured in the New York Times and Washington Post.
In June 2022, the RDB transitioned from its previous hosting location at Medidata to a permanent home with the HHS Technology Group (HTG), to create a repeatable, scalable model for research built off of linked real world data beyond just data related to COVID-19. As part of the transition to HTG, we used Datavant technology to implement the RDB’s linked datasets start-to-finish: for each data source to be linked via Match, we built a separate Segment and used our Remediation Engine to implement the de-identifying operations outlined by Privacy Hub in dataset-specific Expert Determinations. In the past, this suite of remediations could only be implemented via SQL on our technical partners’ side. In the productized version, this happens with a click of a button!
Two years after standing up the COVID-19 Research Database, we’re still just scratching the surface of the potential insights that can be powered by providing academic researchers access to tokenized, linked real world and commercial data sources. Now that Datavant Match is fully integrated into the RDB, all researchers, regardless of their ability to engineer record-matching solutions, have the improved ability to study records within a single cohort and compare records across cohorts. Many of the types of insights that have emerged from the RDB were only pipe dreams a few years ago. These insights have already offered new ways of understanding both our health and our health data.
References
A list of technical partners who made the RDB possible can be seen here:
https://covid19researchdatabase.org/#data
Additional reading on RDB-based studies:
https://covid19researchdatabase.org/blog/
About the Authors
Authored by Aleah Peffer, Isabel Francisco, and Nicholas DeMaison

Aleah Peffer is a Product Success Lead at Datavant where she is a subject matter expert for Match on the Product Success Team. She worked on the COVID-19 Research Database from October 2021 – March 2022 as part of her “Day 1 Project” at Datavant. Connect with Aleah via Linkedin.

Isabel Francisco is a Product Success Lead for the Public Sector vertical within Datavant. The RDB was Isabel’s “Day 1 Project,” in which she focused on researcher support for the COVID-19 RDB. She then managed the platform transition of the RDB to HTG, and continues to work with HTG on new RDB-generated opportunities. Connect with Isabel via Linkedin.
Nicholas DeMaison writes for Datavant, where he leads Talent Branding Initiatives. Connect with Nick via LinkedIn.
Considering joining the team? Check out our careers page and see us listed on the 2022 Forbes top startup employers in America. We’re currently hiring remotely across teams and would love to speak with any new potential Datavanters who are nice, smart, and get things done.